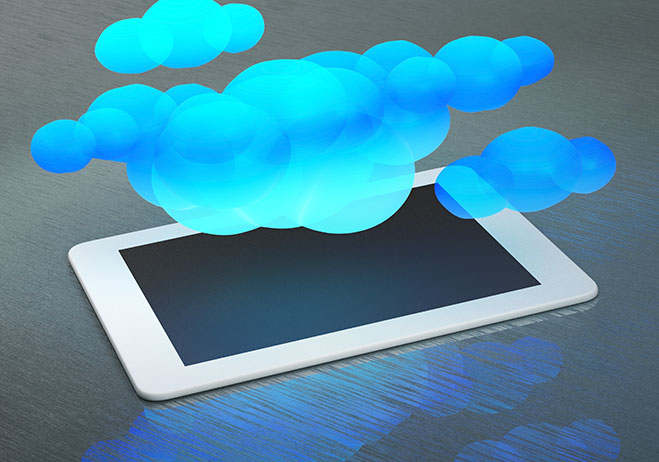
Continued and unerring technological advancements in analytics solutions have made financial institutions consider the possibilities behind big data management. While some firms find themselves mired in floods of near incomprehensible data, others have realised how big data analytics solutions can be used to increase cost-effectiveness and achieving firm-wide risk analysis.
The financial services industry has been increasingly conscious of the gross financial and reputational consequences of having inadequate risk assessment capabilities. The culture of risk management has demonstrated a marked shift post-2008 as numerous companies have since scrambled to protect against further downturns – many having realised the role a big data strategy can play in managing risk processes across the enterprise. Helping to spearhead the sector-wide adoption of cloud technology for risk analytics and big data management is Numerix, a company whose experience in managing OTC derivatives and structured products is second-to-none.
Since the company’s inception in 1996, Numerix has proven a pioneer of analytics for the financial services market. The company’s 200 employees – two thirds of whom possess a PhD or masters degree in either applied mathematics or financial engineering – are highly adept at addressing the various and exacting demands of the complex risk and pricing challenges facing today’s global financial marketplace.
European CEO spoke to the company’s CEO and President, Steven O’Hanlon and CMO, Jim Jockle, about the implications of big data for the financial community and on Numerix’s credentials in offering unparalleled risk and pricing analytics to the market.
How has the recent fascination with big data changed the way organisations do business?
SO: The fascination with big data in the financial community has spurred perhaps the foremost industry development of the past two years and will no doubt continue to do so for the near future.
Stricter regulatory requirements demand that financial institutions have an adequate means of measuring risk, for which increased transparency and big data management is essential. Many and varied asset classes each produce a comprehensive set of data, the likes of which are stored independently and remain a challenge to assess and aggregate when lacking a consistent analytics solution. The lack of consistency, not only across independent silos of data but also across models and measures, has severely hampered the industry’s ability to assess risk in the past.
JJ: At the onset of the financial crisis, individuals were left deciphering spreadsheets trying to calculate risk exposure, as most were without a better means of assessing data holistically. Solutions to help manage this big data and consistency challenge have since proven an incredibly valuable resource in risk management, wherein stringent regulations have come to demand the utmost standards of proficiency and transparency.
Do you think we’re getting a better grasp of risk management?
SO: We’d like to think that the financial community has come to better understand risk. In the past, companies have found it difficult to arrive at pricing and risk calculations quickly and accurately because of the underlying analytics – they’ve had a limited number of models and methods or have needed to build models for clients on an individual basis.
This process is incredibly cumbersome, meaning that the deployment of the risk solution becomes a lengthy process, by which time the marketplace could have changed drastically. We see that the most important underlying aspect to solving any analytics problem is to have an array of models that are industry standard, that can be leveraged through a benchmarking process.
JJ: We are seeing a change in the financial technology mindset. In much the same way you think of Salesforce.com and Apple in terms of the AppStore, we intend to integrate risk into a common and comprehensive architecture. We seek to offer a turnkey solution that can be applied to a multiplicity of circumstances, rather than a heavyweight install system, that can be integrated into the core of a company’s system. By implementing a best-of-breed modular approach to risk analytics, we hope to transform the financial sector from a laggard to a pioneer of big data analytics solutions for risk.
What can cloud technology bring to risk analytics?
SO: We’ve observed a marketplace that has been shifting for some time now, as different institutions have entirely different requirements and capabilities for analysing risk. Institutions with over $1bn under management are usually self-sufficient, deploying their own IT infrastructures and having a tech department to manage those systems accordingly. These companies tend to look for a software resident risk offering to deploy on their investments. However, those with under $1bn under management are by-and-large looking to leverage pre-existing infrastructure and data, in essence doing away with any software or hardware purchases. A cloud solution for big data management allows those without bank-equivalent financial capabilities to operate an equivalent system without the implicated costs of installment and maintenance.
JJ: When we think big data we must also think big compute. When we talk software-as-a-service we inevitably think of data storage, but we must also consider the availability of all these cores for compute purposes. When think of complex issues like the calculation of counterparty credit risk – banks face this compute barrier. Trading operations combined with simulation technology for future exposure understanding produces millions of outputs, and in order to have business impact those must be calculated in real-time. With cloud, end users can utilise cores in the most meaningful way possible, while successfully achieving performance benchmarks.
How can enterprise data management enhance transparency?
SO: An institution’s capacity for transparency ultimately boils down to reporting tools and solutions. Numerix’s analytics architecture provides a flexible and transparent framework within a single analytics platform that can scale to meet companies’ needs, from standalone desktop installs to enterprise-wide deployments enabling an incredibly effective means of accessing and extracting data from an abundance of sources. First and foremost, we maintain a proven ability to produce accurate risk and pricing calculations that users can extract information from and furthermore construct meaningful reports around adhering to government regulations.
JJ: We believe that transparency is not only determined by accurate data but by consistent analytics models. To better illustrate my point, US and European storm tracking conclusions vary wildly as each source utilises a slightly different tracking model. Similarly, as the market changes so too does performance, so you not only need transparency of data, but full understanding of the models’ predictability. You can have the best enterprise data strategy, but if you don’t have the model strategy to go with it you’re missing half the equation.
What’s the best way to deal with large amounts of data?
SO: For companies to differentiate their services in a vastly competitive market they will need to recognise the opportunities to be had in understanding big data implications. We recommend that companies have a road map, outlining the ways in which they wish to capitalise on better big data management. At Numerix we believe the best trading decisions require the best analytics. Through cloud enablement users can scale calculations instantly gaining access to faster processors and more memory without the cost – better aligning pricing and risk across the company.
JJ: The extent to which companies are able to deal with big data is largely determined by the company culture. Whereas regulation sets the basis by which big data is disclosed, the institutions that foster their own culture and infrastructure of risk management are those who will likely avoid the gross financial implications reminiscent of the past.